We’re living in the age of general-purpose AI – large language models (LLMs) and foundation models capable of tasks ranging from writing marketing slogans to generating computer code. Impressive as they are, they rarely reach expert-level performance in any specific area. The saying “jack of all trades, master of none” rings true. But in the rush to create a perfect, all-knowing AI, many businesses are missing a crucial point: this imperfection presents an opportunity for immediate, practical improvements, particularly in industries like insurance. This isn’t about replacing human expertise or achieving futuristic levels of personalisation overnight; it’s about intelligent augmentation – using AI to make existing processes smarter, faster, and more efficient, today.
“A jack of all trades is a master of none, but oftentimes better than a master of one.”
– Originally coined in the 1500s, we could say that this phrase takes on new meaning in today’s AI-driven world.
Why Now?
The combination of readily available AI models, easier fine-tuning, and a growing acceptance of the “good enough” approach is driving rapid adoption. The cost of experimenting has fallen dramatically, and the potential Return on Investment (ROI) is becoming clearer, even for initial, incremental implementations.
First Steps Towards Intelligent Augmentation: A practical Approach
The promise of AI in insurance is exciting, but a complete overhaul of existing systems isn’t realistic for most companies overnight. Instead, the real breakthrough lies in taking incremental steps that leverage the strengths of general-purpose AI, while acknowledging its limitations. Here’s a practical way to get started with intelligent augmentation:
- Identify high-impact, low-risk use cases: focus on areas where AI can enhance existing processes without causing major disruption.
- Rather than overhauling entire functions, organizations can take a more targeted, practical approach to AI adoption.
- Underwriting doesn’t need to be completely re-engineered or replaced. A more effective first step is to enhance current processes by integrating AI tools that can flag potentially high-risk applications for further human assessment. For example, AI can scan submissions for anomalies or unusual data patterns, prompting human underwriters to take a closer look.
- In customer service, instead of fully replacing representatives with chatbots, companies can introduce AI-driven chatbots to manage high-volume, routine queries—such as resetting passwords or updating contact details. This allows human agents to focus on more nuanced or sensitive customer issues. It’s essential to train these bots thoroughly for specific tasks and actively monitor their performance, with human support stepping in when needed.
- For claims handling, the goal isn’t to automate the entire department from day one. A more manageable starting point is optimizing the First Notice of Loss (FNOL) process. This could involve using AI to analyze images of damage and provide immediate feedback to customers about possible next steps, accelerating the initial phase of the claims journey.
- Prioritise data quality and availability: remember the adage: “Garbage in, garbage out”. AI models are only as good as the data they’re trained on.
- Embrace iterative development and fine-tuning
- Rather than striving for a flawless AI solution right from the start, it’s more effective to adopt a “minimum viable product” (MVP) mindset. Begin with a simple, functional version of the AI system and improve it over time through real-world testing and user feedback. Techniques like A/B testing can help compare different model configurations to determine what works best. This incremental, data-driven approach reduces risk while enhancing return on investment.
- For example, you might launch a chatbot designed to address the 10 most frequent customer inquiries. Monitor its accuracy and effectiveness, pinpoint areas where it falls short, and fine-tune it with better data and responses. As its reliability increases, you can gradually expand its scope and capabilities.
- Focus on human-AI collaboration
- Key Principle: AI should enhance human capabilities, not replace them entirely.
- Implementation: Design workflows that seamlessly integrate AI tools with human expertise. For example, an AI might pre-process claims data, highlighting key information and potential fraud indicators, but a human claims adjuster makes the final decision. This ensures accuracy, fairness, and accountability.
- Example: AI can identify key information and sentences in a document and point users to them; however, a human is still needed to make the best decisions.
- Establish ethical guardrails
- Recognise: AI systems can perpetuate biases present in the data.
- Action: Implement rigorous testing and monitoring to ensure fairness and prevent discrimination. Establish clear guidelines for data privacy and security. Be transparent with customers about how their data is being used and how AI is involved in decision-making.
Rethinking AI: From Perfection to Practical Enhancement
The “Jack of all Trades” nature of current AI isn’t a weakness; it’s the key to unlocking immediate, practical improvements. By embracing fine-tuning, starting with manageable steps, and focusing on specific business needs, insurers can leverage general-purpose AI to improve efficiency, support their staff, and gain a competitive advantage. Start small, iterate quickly, prioritise ethics, and augment human expertise with “good enough” AI. The time to act is now.
At Ivy Partners, we believe that delivering lasting success requires combining deep expertise with a commitment to these core principles. If you’re ready to explore how intelligent augmentation can accelerate your digital transformation, we’re here to help.
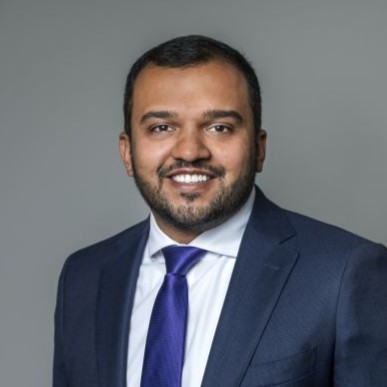
About the Author
Hamad Masood is a Principal Consultant at Ivy Partners, based in Zug, Switzerland. He brings extensive experience in delivering strategic guidance and innovative solutions within the insurance industry. Recognized as a leader in the field, Hamad plays a key role in driving organizational growth and enhancing operational efficiency through his deep expertise in business analysis, digital transformation, and strategic planning.