As someone born in the 90s, I grew up with a growing awareness that every choice—big or small—has an impact on the planet. From school recycling campaigns to early environmental movements, sustainability became a core value for many in my generation.
Now, as an engineer in the tech industry, I find myself at the heart of innovation. I’m constantly inspired by the breakthroughs we’re making with AI—the potential to solve problems faster, smarter, and at scale is genuinely thrilling. It’s a privilege to contribute to technologies that can improve lives and reshape entire industries.
But with that privilege comes responsibility.
Behind the algorithms and infrastructure, there’s a real cost: energy, resources, and environmental impact. And while I celebrate the power of AI, I also feel a growing need to question how we build it—and at what cost. That’s where purpose comes in.
To me, progress with purpose means ensuring that technological advancement doesn’t come at the planet’s expense. It means building systems that are not only smart but sustainable. It means being just as intentional about how we power innovation as we are about what we innovate.
Because in the end, true progress is not just about what we create—it’s about what we preserve.
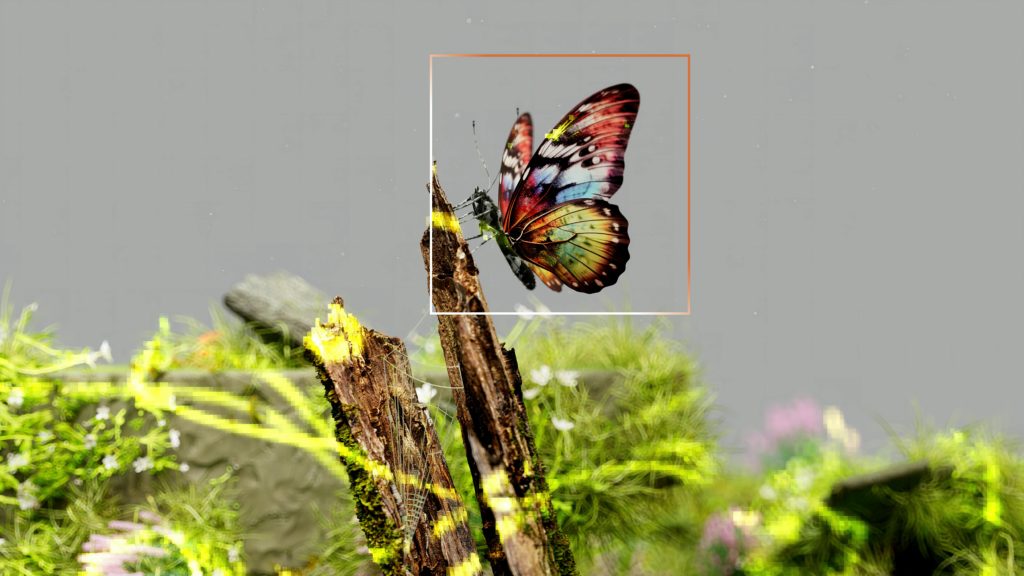
©️ Google DeepMind
1. The Environmental Costs of AI
The development of AI models—particularly large-scale neural networks—demands vast computational resources. Training these models requires intensive computation, which in turn leads to significant energy consumption and, consequently, higher carbon emissions. Data centers powering AI systems often rely on electricity sourced from non-renewable energy, exacerbating environmental degradation.
One often overlooked aspect is the short life cycle of generative AI models. Companies are releasing newer, often larger models at an accelerating pace—sometimes every few weeks. As a result, the considerable energy consumed to train previous models is quickly rendered obsolete. Even more concerning is the volume of training and testing iterations that never make it to production. These experimental phases still consume massive amounts of energy, adding to the hidden costs of AI’s development pipeline.
Compounding the issue is AI’s rapid democratization. No longer confined to data scientists and engineers, large language models (LLMs) like ChatGPT and Gemini are now being used by millions of non-technical users every day. According to MIT News (Jan. 2025), a single ChatGPT query consumes up to five times more energy than a standard web search. When scaled across global usage, the energy demand becomes staggering.
There’s no doubt that AI is transforming industries—healthcare, finance, transportation, and beyond. But behind the promise lies a pressing and often underestimated concern: the environmental cost of innovation at scale. As AI tools become more accessible and integrated into everyday life, so too does the responsibility to understand—and mitigate—their energy demands.
Each AI helper runs within the client’s cloud tenancy, begins as a pilot, and scales only with proven time savings.
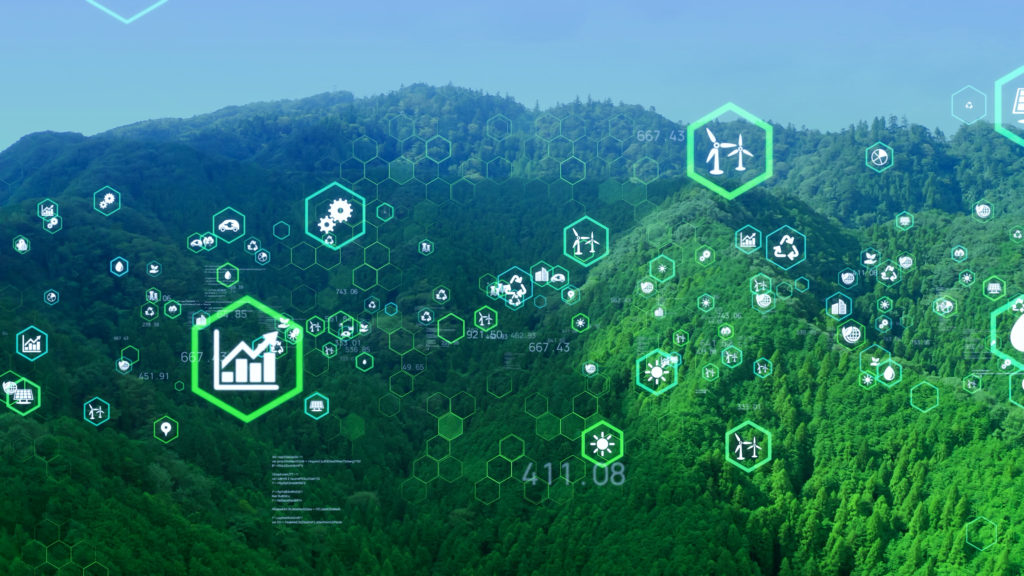
©️ Metamorworks
2. Green AI: A Paradigm for Sustainable Innovation
In response, Green AI has emerged—not as a trend, but as a necessity. It calls for efficiency, transparency, and ecological responsibility at every stage of AI development and deployment. It’s about optimizing not just for accuracy or scale, but also for energy usage and emissions.
By improving processes such as fuel consumption, failure prevention, and predictive maintenance, AI enables power plants to operate more reliably and with lower emissions.
These optimizations not only help reduce costs but also minimize the environmental footprint of an industry traditionally associated with high levels of pollution. Several initiatives and AI applications have been and continue to be launched to address these challenges:
- Earth Friendly Computation: Pioneered by genome scientists Keolu Fox and Eric Dawson, this initiative integrates sustainable practices into computational science. It combines Indigenous knowledge systems with modern computational methods to reduce the environmental impact of large-scale data processing. A notable project involves repurposing discarded GPUs to minimize electronic waste, extending the lifecycle of technological hardware, and lowering the carbon footprint associated with AI development. Earth Friendly Computation: Applying Indigenous Data Lifecycles in Medical and Sovereign AI
- Destination Earth (DestinE): An initiative by the European Commission, DestinE aims to create a high-precision digital simulation of Earth. This digital twin will utilize real-time data to better understand climate change effects and environmental disasters, aiding policymakers in crafting effective responses. By integrating vast datasets, DestinE exemplifies how AI can be harnessed for environmental monitoring and sustainability.
- AI Action Summit: In February 2025, the AI Action Summit brought together representatives from 58 countries to discuss the future of AI. A key outcome was the “Statement on Inclusive and Sustainable Artificial Intelligence for People and the Planet,” emphasizing the development of AI that is open, transparent, ethical, safe, and trustworthy. The declaration also highlighted the need to avoid market concentration in AI development to encourage innovation and promote sustainability.
- Green AI Institute: A collective of visionary researchers, academics and professionals dedicated to advancing the integration of artificial intelligence and environmental sustainability. Some of the key research areas include energy-efficient AI algorithms, sustainable data center operations and ethical implications of AI in sustainability. Green AI Institute
- Model Efficiency: Researchers are developing more efficient AI architectures to reduce computational requirements without compromising performance. For example, models like BLOOM have been trained using nuclear energy and with more efficient chips, releasing only 25 metric tons of CO₂ compared to others.
- Renewable Energy: Tech companies are investing in renewable energy sources to power data centers. Google’s partnership with Kairos Power to build small modular nuclear reactors aims to meet the rising energy demands of its AI data centers with carbon-free power.
- Prompt engineering: Earlier this year, Google released a comprehensive 68-page guide outlining Best Practices for Prompt Engineering when working with large language models (LLMs). One of the key insights from the document is that longer model outputs significantly increase both the computational cost and the associated energy consumption. This highlights an important, often overlooked aspect of prompt design: its environmental impact. By following these best practices, developers and researchers can not only improve the quality and relevance of model responses but also reduce the resource demands of their applications. In doing so, prompt engineering becomes not just a technical skill, but a responsible approach to sustainable AI usage. The guide serves as a valuable resource for anyone looking to write more effective and efficient prompts.
- Energy-Efficient Buildings: AI technologies are optimizing energy consumption in buildings. For example, AI-driven systems have been implemented to reduce HVAC energy usage, leading to significant cost savings and carbon emission reductions.
- Renewable Energy Deployment: Companies like Terabase Energy are utilizing AI and robotics to accelerate the construction of large-scale solar farms. By automating assembly processes, these technologies enhance efficiency and reduce the time required to deploy renewable energy solutions.
- Carbon Capture Materials: AI is aiding in the design of new materials for carbon capture. For instance, Amazon is piloting an AI-designed material aimed at removing carbon emissions from its data centers, addressing the environmental impact of AI infrastructure.
- Carbon Accountability: Tools like CodeCarbon enable developers to measure the carbon footprint of their code, promoting awareness and encouraging optimization to reduce emissions.
- Optimization in Energy Industry: AI is increasingly being used to optimize energy production in ways that significantly benefit both operational efficiency and the environment. By improving processes such as fuel consumption, failure prevention, and predictive maintenance, AI enables power plants to operate more reliably and with lower emissions. These optimizations not only help reduce costs but also minimize the environmental footprint of an industry traditionally associated with high levels of pollution. As energy systems become more complex and demand more sustainable solutions, AI stands out as a powerful tool for driving cleaner, smarter, and more efficient power generation.
3. Challenges & Future Directions: A Responsibility we Can’t Ignore
While progress has been made, several significant challenges persist in the pursuit of Green AI. Energy consumption remains a primary concern—despite recent advancements, training and deploying AI models is still highly resource-intensive. Reducing this footprint will require continued development of more energy-efficient algorithms, hardware, and infrastructure.
Equally important is the careful allocation of resources: balancing the ambition to advance AI with the urgent need to conserve our planet demands thoughtful planning and bold policy interventions.
International collaboration is also essential, as the path to sustainable AI cannot be achieved in isolation. Establishing global standards, sharing best practices, and ensuring equitable access to green technologies will be critical. At the same time, hardware innovation must accelerate—particularly in designing low-power chips and AI accelerators for edge computing and mobile applications. Policy and regulation can further guide this transition by introducing incentives for sustainable practices or placing limits on the energy consumption of large-scale AI operations.
Among these challenges, one stands out as especially urgent and often overlooked: the need for widespread AI literacy. As AI becomes embedded in our daily lives and across industries, understanding its mechanisms and consequences must go beyond technical expertise. There’s a pressing need for greater awareness of AI’s ethical dilemmas, societal impact, and environmental footprint. Building this awareness—through education, public engagement, and transparent communication—is key to fostering a more responsible, informed society. Articles like this aim to contribute to that goal. A collective understanding of both the promises and risks of AI is essential for navigating its evolution responsibly, and ensuring it delivers outcomes that genuinely benefit humanity.
Green AI offers a roadmap, prioritising energy efficiency, transparency, and responsible development. It represents a critical intersection between technological innovation and environmental stewardship. By acknowledging and addressing the ecological impacts of AI, the global community can harness its potential while safeguarding the planet for future generations. Ongoing research, collaborative initiatives, and a commitment to sustainability are essential to realizing the vision of an environmentally friendly AI landscape.
At Ivy Partners, we believe technology should not just be smart—it should be wise. That means designing and implementing solutions that are not only high performing, but also ethically and ecologically sound. The future of AI must be one where innovation and environmental stewardship go hand in hand. We don’t need to wait for permission to act – we need to lead with purpose, responsibility, and vision. The path to sustainable AI is ours to shape. Let’s begin.
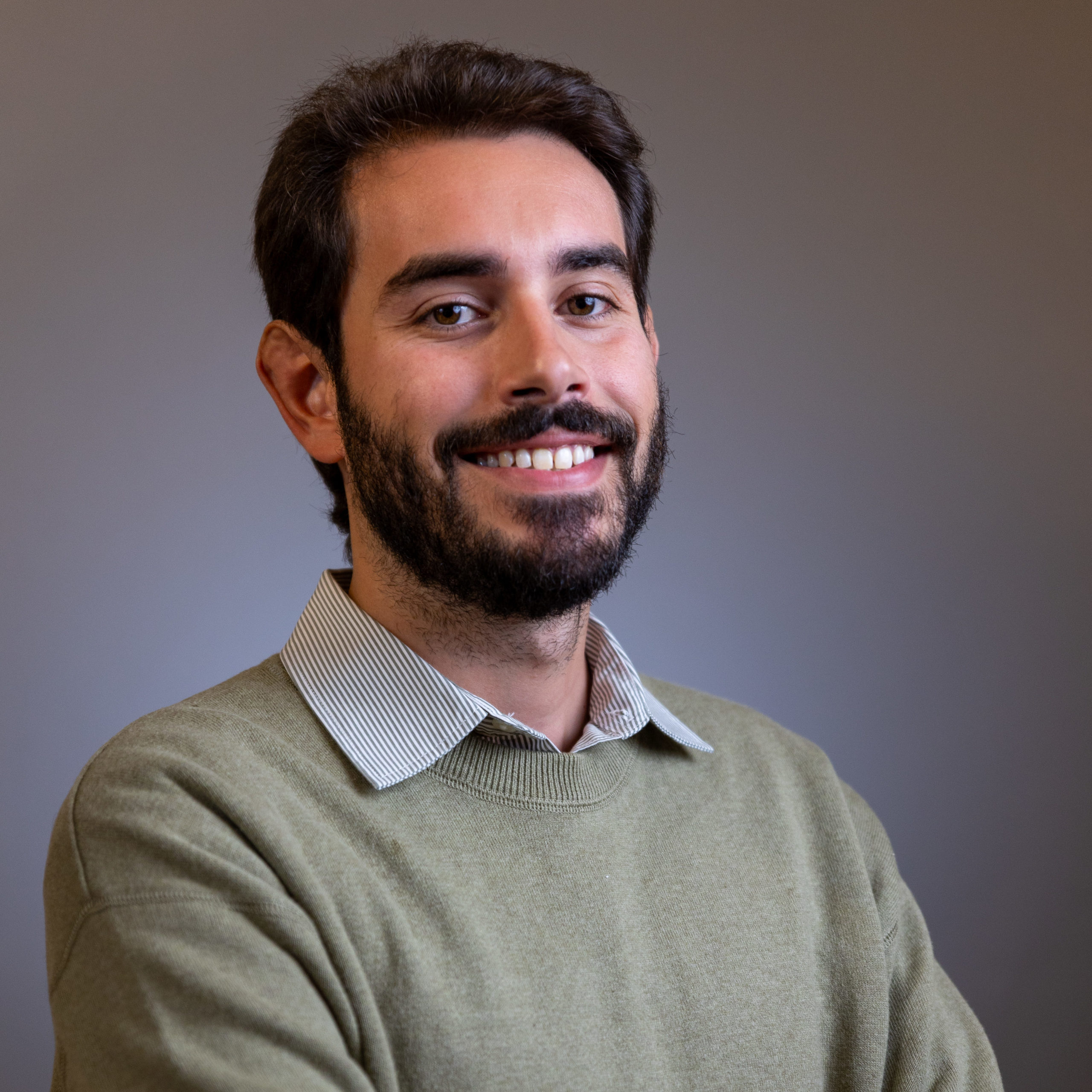
About the Author
Ricardo Sousa
Ricardo Sousa is Data Scientist at Ivy Partners. Over 4 years of experience applying Machine Learning and data analytics to drive decision-making in the energy and insurance industries. With a strong background in statistical modeling, predictive analytics, data engineering and data visualization, Ricardo has worked on projects ranging from optimization of energy efficiency in data centers to optimization of power plants and claims automation. Passionate about using data and statistics for impact, Ricardo brings a practical, results-oriented approach to solving complex business challenges through AI and data-driven solutions.